This is a Customer-Churn prediction project incorporated with NLP technologies and also machine learning models.
The core purpose of this study is to find the impact of Sentiment Analysis in predicting customer churn for the e-commerce industry by employing different predictive models. Furthermore, the study is also focused on observing which model is best in a more accurate prediction for determining the churn rate of customers.
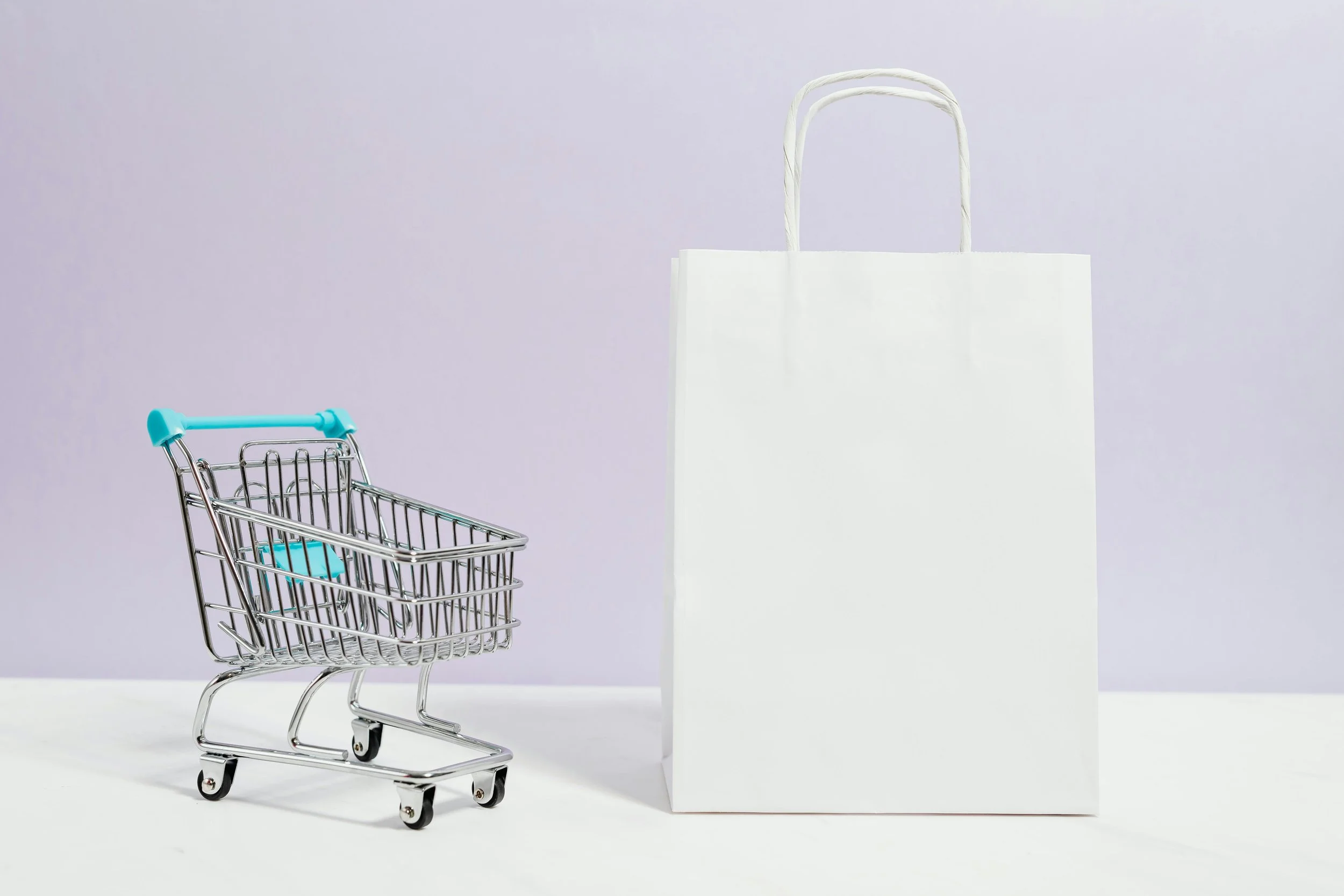